by Team ProcessMiner | Mar 19, 2024 | Insights, Wastewater
The wastewater treatment sector is facing a significant regulatory shift. Stricter laws and regulations have increased the pressure on wastewater treatment plants to adapt and evolve.
Recent amendments in EPA guidelines focusing on nutrient removal have highlighted the urgency for wastewater treatment plants (WWTPs) to refine their processes and serve as a testament to that shift.
This landscape of tightening regulations, combined with traditional wastewater management challenges, makes it clear that innovation is key to achieving compliance and operational efficiency.
Factors Driving The Need for Innovation in Wastewater Treatment
The urgency for innovation in wastewater treatment has never been greater, driven by a confluence of critical factors:
- Rising Microplastics: The increase of microplastics in water sources is alarming. Studies have shown that conventional treatment processes often fail to fully remove these contaminants, posing a risk to both human health and the environment. This underscores the need for advanced wastewater treatment technologies for removing finer particles.
- Escalating Pollution Levels: Pollution in water bodies is escalating at an unprecedented rate. The World Health Organization reports that over 80% of the world’s wastewater is discharged without adequate treatment, leading to severe environmental and health impacts. Innovative treatment methods are crucial to address this escalating issue.
- Population Growth and Urbanization: The world’s population is expected to reach 9.7 billion by 2050, with a significant portion residing in urban areas. This demographic shift increases the strain on existing wastewater infrastructure, demanding more efficient and scalable treatment solutions.
- Financial Implications of Non-Compliance: The financial burden of failing to meet regulatory standards is substantial. For instance, in the United States, non-compliance with EPA regulations can result in fines of up to $25,000 per day. This highlights the economic imperative for WWTPs to adopt innovative, compliant technologies.
- Increasing Energy Demands: Wastewater treatment is energy-intensive, accounting for a significant portion of municipal energy use. Innovations aimed at reducing energy consumption can lead to substantial cost savings and environmental benefits.
- Global Focus on Sustainable Practices: There is a growing global emphasis on sustainable and eco-friendly practices. Adopting innovative wastewater treatment methods aligns with this trend and enhances a facility’s reputation for environmental stewardship.
These factors collectively emphasize the critical need for an advanced AI-driven approach to wastewater treatment. One particular area that has a transformative effect in enabling great compliance and sustainability is the sludge dewatering process.
Innovating Wastewater Treatment Starts with Sludge Dewatering
As sludge dewatering is one of the highest contributing costs to wastewater treatment, it represents an ideal place to integrate advanced technology with traditional processes. This crucial step, integral to the treatment cycle, is ripe for a technological overhaul.
Traditional reactive and manual methods often fall short in efficiency and compliance. Improvements in this area enhance the overall treatment process and play a pivotal role in meeting regulatory standards.
The integration of AI and Machine Learning emerges as a new path to enhanced precision in polymer dosing and greater efficiency in the sludge dewatering process. AI-driven solutions can significantly reduce costs and enhance compliance by optimizing polymer dosing. As an example, ProcessMiner’s advanced AI solutions for wastewater treatment are a practical example of this leap forward, offering a combination of operational efficiency and regulatory adherence previously unattainable.
By optimizing processes like polymer dosing and enhancing overall treatment efficiency, AI in wastewater treatment addresses these challenges and paves the way for sustainable, compliant, and cost-effective operations. To learn more about cost-efficiencies that can be obtained by optimizing the sludge dewatering process with AI, download our guide, “The High Costs of Inefficiency: Optimizing Wastewater Treatment.”
by Team ProcessMiner | Mar 8, 2024 | Insights, Wastewater
Governments and environmental agencies worldwide increasingly recognize the urgent need to tackle water-related issues. For instance, the EPA emphasizes the critical role of Wastewater Treatment Plants (WWTPs) in mitigating these challenges.
With the advent of AI and Machine Learning, there’s a promising pathway to navigate these issues more effectively. But what exactly is driving the need for more advanced wastewater treatment solutions?
Understanding the Need for Advanced Wastewater Treatment Solutions
WWTPs are at the frontline of environmental challenges, notably:
Emerging Technologies in Wastewater Treatment
Advanced wastewater treatment technologies, particularly AI in sludge dewatering, are pivotal. By optimizing operations, AI systems enhance efficiency and reduce costs, addressing environmental and operational challenges.
The Importance of Sustainable Practices in Wastewater Management
Beyond addressing immediate environmental challenges, advanced wastewater treatment plays a long-term role in preserving ecosystems. Implementing these methods contributes significantly to the larger environmental preservation and sustainability goal.
For example, by employing waste-to-energy technologies, wastewater facilities treat water and harness renewable energy, reducing the overall environmental impact. This holistic approach to wastewater management is crucial in maintaining the ecological balance and ensuring a healthier planet for future generations.
Regulatory Compliance and Its Impact on Wastewater Treatment
Staying updated with environmental regulations is crucial for wastewater treatment facilities. These rules, which constantly evolve, dictate how facilities should operate. Adapting to these changes often means upgrading to advanced treatment technologies.
These upgrades help meet stricter standards, avoid penalties, and enhance the facility’s reputation. Ultimately, keeping pace with regulations ensures sustainable and legally compliant operations in wastewater treatment.
Tackling Environmental Challenges With Advanced Wastewater Treatment
The world faces various environmental challenges, each more pressing than the last. Climate change is accelerating, and water scarcity is becoming a more significant crisis day by day. To combat these issues, innovative and sustainable approaches are essential.
Advanced wastewater treatment emerges as a key player in this fight, offering effective and efficient solutions to some of our time’s most pressing environmental concerns.
Maximizing Environmental Preservation Through Advanced Wastewater Treatment
Advanced wastewater treatment represents a significant leap beyond traditional methods, employing the latest technologies and processes to remove harmful substances and pathogens from wastewater. This elevated approach is crucial for ensuring water’s safe discharge or reuse, playing a pivotal role in environmental preservation.
These advanced systems do more than just comply with rigorous regulatory standards; they actively promote environmental sustainability. By reducing water pollution and encouraging water conservation, advanced wastewater treatment becomes a cornerstone in our efforts to protect and sustain our natural world.
Leveraging Advanced Wastewater Treatment for Strategic Business Advantages
Investing in advanced wastewater treatment systems is a strategic and forward-thinking choice for industry leaders and decision-makers. This decision extends beyond simply adhering to environmental regulations; it positions early adopters as leaders in environmental responsibility.
Furthermore, the long-term economic benefits of such an investment are significant. Implementing advanced treatment methods and reusing wastewater can substantially reduce water usage, cutting down operational costs.
This not only contributes to the facility’s bottom line but also aligns with sustainable business practices, creating a win-win scenario for both the environment and the water authority.
Empowering Wastewater Treatment Plant Operators: Key Players in Environmental Solutions
Wastewater treatment plant operators are central to the effectiveness of advanced wastewater treatment systems. Their expertise is vital in maintaining the efficiency and ensuring the compliance of these treatment processes.
The ability of operators to adeptly manage and utilize these sophisticated systems enhances operational effectiveness and efficiency. As a result, their role is critical in addressing and overcoming environmental challenges, positioning them as key contributors to the field of environmental sustainability.
Future Outlook: The Evolution of Wastewater Treatment
As we look toward the future, wastewater treatment is set to undergo significant evolution, driven by technological advancements and the growing need for sustainable practices. The integration of cutting-edge technologies like artificial intelligence (AI) and machine learning (ML) is poised to revolutionize this field.
Artificial Intelligence and Machine Learning
The application of AI and ML in wastewater treatment is particularly promising. These technologies can be used for predictive maintenance, which anticipates equipment failures before they occur, thereby reducing downtime and maintenance costs. Furthermore, AI and ML can optimize operations by analyzing patterns in data, leading to more efficient treatment processes and better resource management.
Advancements in Treatment Technologies
Future advancements are also expected in the treatment technologies themselves. Innovations in filtration methods, chemical treatments, and biological processes will continue to improve the efficiency and effectiveness of wastewater treatment. These advancements will enable facilities to handle larger volumes of wastewater and treat it to higher standards, which is crucial as urban populations continue to grow.
Sustainability and Resource Recovery
Another key trend is the shift toward sustainability and resource recovery. Future wastewater treatment processes will likely focus on treating water and recovering valuable resources from wastewater, such as energy, nutrients, and even potable water. This approach aligns with the global move toward circular economies, where waste is minimized and resources are reused.
Adapting to Climate Change
Finally, the impact of climate change will necessitate adaptive strategies in wastewater management. This includes designing systems that can withstand extreme weather events and incorporating treatment processes that reduce greenhouse gas emissions.
The future of wastewater treatment is marked by technological innovation, a stronger emphasis on sustainability, and a responsive approach to global challenges like urbanization and climate change. These developments are not just transforming the industry but are also crucial in ensuring a sustainable future.
To learn more about this topic, read our blog on how AI is driving innovation and compliance in wastewater treatment here.
by Team ProcessMiner | Mar 1, 2024 | Insights, Wastewater
Achieving the right balance in polymer dosing remains a nuanced and complex task for wastewater treatment professionals. This dynamic process is significantly influenced by the constantly changing nature of wastewater inflow and composition. Traditional manual dosing methods, while critical, are prone to human error and often lead to over- or under-dosing.
Leveraging AI-Driven To Obtain Precise Polymer Dosing
Modernizing the polymer dosing process by integrating advanced technology like AI can be transformative. AI allows for a more precise and dynamic process tailored to the specific and real-time characteristics of the individual plant. In addition, by automating polymer dosage, plant managers can minimize human error and free up staff to focus on other daily activities.
Overcoming Polymer Dosing Challenges with Expertise and Innovation
Today’s reliance on manual dosing methods is a visual and inconsistent trial-and-error approach, which, while practical, leads to a lack of consistency and precision required for optimal sludge treatment.
Combining wastewater professionals’ domain expertise with AI represents an effective combination of experience, expertise, and data analytics. Leveraging historical knowledge of the intricacies of the treatment process with insight from process data to make timely enables 24/7 adjustments to the application of polymer.
Refining the Polymer Dosing Process
In an industry where efficiency and compliance cannot be compromised, refining the polymer dosing process is not just about following a static formula. It’s about adapting to the ever-changing nature and complexity of wastewater treatment to find the most effective, sustainable, and cost-efficient approach on an ongoing basis.
AI’s Predictive Power in Wastewater Treatment
Deploying operational solutions that combine AI technology with intelligent sensing devices stands out as a modernization strategy. This approach is perfectly designed to monitor and accurately predict water treatment needs and make real-time adjustments for optimized operational results. Beyond mere data processing, this strategy ensures optimal polymer dosage, minimized energy consumption variance, and consistent production of a drier sludge cake.
These insights enable treatment plants to proactively adjust their processes, shifting from a traditionally reactive execution to a proactive strategy. With AI’s guidance, chemical dosing becomes more responsive to the current conditions of the wastewater, allowing plants to maintain consistent operational efficiency and adapt quickly to changes.
4 Benefits of AI in Wastewater Polymer Dosing
Introducing AI into wastewater treatment, particularly in polymer dosing, marks a significant leap forward. The benefits are quantifiable and multifaceted, impacting critical aspects of the treatment process.
1. Enhanced Treatment Efficiency
By ensuring precise dosing, AI technology can eliminate polymer waste and shortfalls. This level of accuracy not only translates to significant cost savings, but also boosts the overall efficiency of the treatment process.
For example, an AI-supported polymer dosage system can reduce operating costs for various types of sludge dewatering. Moreover, AI models have shown excellent prediction and optimization performances in wastewater treatment, including polymer dosing. AI enables wastewater treatment plants to hit that sweet spot in polymer usage every time, leading to more sustainable and efficient wastewater treatment processes.
2. Minimized Environmental Impact
In 2021, facilities in the United States released 196 million pounds of chemicals into water bodies, with nitrate compounds accounting for 90% of these releases, often as a result of on-site wastewater treatment processes. Globally, more than 80% of wastewater returns to the environment untreated, contributing to pollution and eutrophication.
AI-driven wastewater treatment can significantly mitigate these issues. By leveraging precise dosing, AI ensures fewer chemicals escape the environment. This meticulous management of chemical use aligns with stringent environmental regulations and represents a leap toward more sustainable wastewater practices. AI’s ability to optimize chemical usage reduces the risk of chemical leaks, thereby diminishing the ecological footprint of wastewater treatment and safeguarding our water resources.
3. Reduction in Manual Labor and Human Error
Human error in wastewater treatment can have significant consequences. For example, sewage treatment plants on the Ganga were found to be operating at less than 60% of their installed capacity due to issues such as human error. This inefficiency can lead to the release of harmful pollutants into the environment.
However, using AI-driven systems in wastewater treatment presents a promising solution to this pressing issue. By automating the monitoring and adjusting involved in polymer dosing, AI technology reduces the workload on staff and significantly lowers the chances of human error. Reducing human error is critical in maintaining consistent water treatment quality and can lead to more sustainable and efficient wastewater treatment processes.
4. Data-Driven Insights for Improved Decision-Making
One of the most significant advantages of AI is its ability to analyze data and provide previously inaccessible insights. These insights enable wastewater treatment professionals to make more informed decisions and fine-tune the treatment process for better results. This aspect of AI is more than just efficiency. It’s about empowering professionals with knowledge for continuous process improvement.
Incorporating AI into wastewater treatment isn’t just a technological upgrade; it’s a strategic move toward smarter, more sustainable operations. It represents a shift in how the wastewater industry approaches challenges – with intelligence, foresight, and a focus on long-term sustainability.
Embracing AI in Wastewater Treatment for a Sustainable Future
Integrating AI-driven strategies, especially polymer dosing, signifies a significant shift toward a sustainable future. This move goes beyond merely keeping up with technological advancements. It represents a deep commitment to eco-friendly and efficient wastewater management practices. As the industry grapples with ever-tightening environmental regulations, a diminishing workforce, and a growing emphasis on sustainability, AI emerges as a crucial ally.
AI’s role in wastewater treatment is becoming more critical by the day. It offers a way to meet stringent environmental standards while streamlining operations. The technology brings precision and efficiency that traditional methods can’t match, enabling facilities to minimize waste, reduce costs, and lessen their environmental impact.
Interested in learning more? Download your copy of our infographic to learn how AI works in the sludge dewatering process.
Get the Infographic
by Team ProcessMiner | Mar 1, 2024 | Insights, Wastewater
Over the years, more effective wastewater treatment methods have significantly reduced sludge cake volume. Today, the average volume of sludge is just 1% of influent wastewater treated, which drops to 0.5% with dewatered sludge. However, even with these relatively small volumes, sludge cake management can still lead to a massive drag on a wastewater treatment plant’s bottom line.
Between 40-60% of plant costs can be attributed to the handling of sludge. Current environmental regulations are more stringent than ever and can be expected to become even more so as population growth and urbanization continue to increase the strain on wastewater treatment systems. Both transportation and chemical costs are climbing, and options for disposal are becoming more limited. Sludge cake management expenses, therefore, need to be streamlined for plants to remain cost-effective.
Thankfully, new technologies provide solutions to help plant managers operate more efficiently. Advanced Artificial Intelligence (AI) tools are becoming the gold standard for monitoring metrics and increasing the efficiency of the sludge dewatering process, leading to reduced costs and improved regulatory compliance.
Sludge Cake: The Weight of the Issue
In the early 20th century, there was a worldwide drive to clean wastewater discharge before releasing it into the environment. Since then, increasingly stringent regulations have forced wastewater treatment plants to provide more levels of treatment and find new ways to use and dispose of sludge safely.
At the same time, the cost of sludge disposal, transportation, and chemicals (like polymers) have increased. Dewatered sludge cake, for example, can consist of anywhere between 18-35% dry solids, and this variability creates an opportunity for improvement for plant operators.
Check out this white paper to learn more about the cost of wastewater treatment inefficiencies.
There’s also the issue of ongoing variability in sludge densities, impacting operational costs due to the increased frequency of equipment shutdowns and startups. In addition, labor costs are also negatively impacted as man-hours needed to address these situations are increased.
With these pressures, plant managers must identify new solutions that supplement current practices and enhance operational efficiency. With the help of technology, we can consistently reduce the amount of water in sludge cake while optimizing polymer dosage and improving equipment availability.
Embracing AI for a More Efficient Sludge Cake
While wastewater treatment and management has evolved, there is still an opportunity for significant process improvement. According to one study, 58% of wastewater is improperly managed. Current wastewater treatment techniques, natural variation, and inefficiency mean that wastewater treatment plants need help to increase this percentage.
AI is one advanced technology already implemented in many plants for water quality monitoring, equipment energy optimization, and operation simulation. For example, wastewater pumping stations that have deployed AI have seen a 16.7% reduction in electricity consumption. AI can also be effective in monitoring and optimizing the sludge dewatering process.
When integrated with intelligent sensors and meters, AI can proactively predict end-of-process moisture levels and recommend the precise polymer dosage required to meet targeted sludge dryness specifications. And, since this can be executed autonomously, it frees up man hours for other operational priorities.
Using AI in the Sludge Dewatering Process
Clear operational challenges remain within the sludge dewatering process. Although solutions like thermal hydrolysis and enzymic hydrolysis have been effective at breaking down sludge and making it easy to dispose of, these labor-intensive techniques add significantly to bottom line costs.
Despite some modernization, monitoring, and testing are still largely manual operations and prone to human error, leading to under and over-dosing of polymer based on visual analysis of effluent quality. Failing to perfect processes like these can cost hundreds of thousands of dollars for wastewater treatment plants annually, with treatment costing anywhere between $2-18/kgal and escalating annually.
As previously mentioned, AI-assisted processes can monitor plant conditions 24/7, accurately predict inefficiencies in real time, and recommend and even execute corrective action autonomously. This allows for more accurate and reduced polymer dosing and drier sludge cake, directly contributing to reduced sludge transportation or incineration costs.
It has been estimated that even a 1% decrease in sludge cake water content can represent a savings of up to $100,000 annually. In addition, with advanced technology, monitoring becomes an around-the-clock process, and dosage is based on detailed analysis of historical data and output specifications. This ultimately reduces process control variability, improving equipment uptime, and mean time between failures.
Making the Change to Modern Sludge Dewatering Processes
Adopting emerging technologies designed to improve plant operating efficiency is becoming necessary. Keeping pace with the increasing demand for clean water, sustainable solutions, and the desire to streamline costs requires continuing the evolution of the wastewater treatment process. The adoption of technologies like AI, when coupled with traditional best practices, represents a pragmatic modernization step toward ever-increasing efficiency.
Today, integrated turnkey solutions are available that can be fully operational in a couple of months, work seamlessly with existing IT and OT infrastructure, require no special technical staffing, and can be implemented without disrupting day-to-day operations. Through the development of a comprehensive strategy, an approach that includes technological adoption and commitment to continuous improvement can unlock the potential for improved efficiency, quality, and innovation.
To learn more about the future of wastewater treatment and some of the technologies that are already changing the wastewater landscape, read our blog: “AI-Driven Process and Quality Optimization in Modern Wastewater Treatment Facilities.”
by Team ProcessMiner | Jan 24, 2024 | Insights
In the evolving landscape of plastics manufacturing, the integration of deep learning and AI technologies is redefining standards for quality control, operational efficiency, and sustainability. This transformative approach goes beyond enhancing production processes; it’s reshaping the entire industry.
Deep Learning: A Game-Changer in Plastics Quality Control
The implementation of deep learning in plastics manufacturing marks a significant shift from traditional quality control methods. These advanced algorithms excel in detecting even the minutest defects in products, ranging from surface imperfections to structural inconsistencies. For instance, a study by McKinsey found that using deep learning for defect detection in manufacturing can increase accuracy by up to 90%. This level of precision in quality control ensures that every product leaving the production line meets stringent quality standards, thereby significantly boosting customer satisfaction and trust.
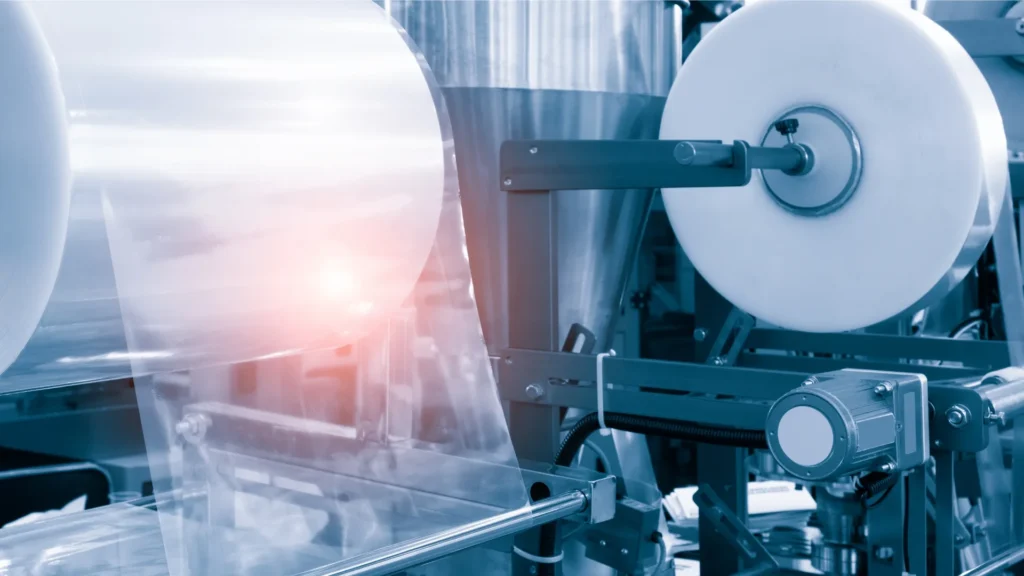
Technical Depth of AI in Manufacturing
The technical aspects of AI, such as machine learning algorithms and data analytics, play a critical role. For instance, Deloitte highlights how AI can optimize supply chain management in manufacturing, balancing stock levels and predicting lead times.
Impact on Plastics Operational Efficiency
Deep learning algorithms, when coupled with real-time data analysis, empower plastics manufacturers to make swift, informed decisions. This agility is crucial in rapidly responding to market demands and fluctuating production dynamics. By accurately predicting potential production issues and swiftly addressing them, manufacturers can significantly reduce downtime and enhance overall plastics operational efficiency.
Predictive Maintenance: Proactive Rather Than Reactive
One of the standout benefits of incorporating AI and deep learning in plastics manufacturing is predictive maintenance. This proactive approach uses real-time data to foresee equipment malfunctions before they occur, allowing for timely maintenance and avoiding costly production halts. This not only extends the lifespan of machinery but also ensures a smooth, uninterrupted manufacturing process.
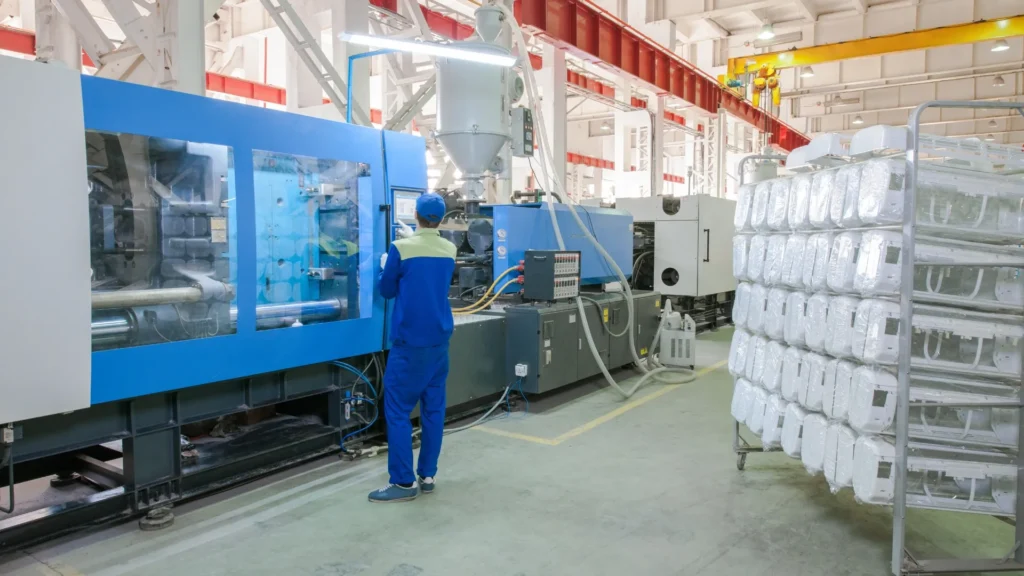
ProcessMiner: Leading the AI Revolution in Plastics Manufacturing
ProcessMiner has emerged as a frontrunner in bringing these AI-driven advancements to the plastics manufacturing sector. Our platform leverages real-time data to provide actionable insights, enabling manufacturers to fine-tune their processes continuously. From reducing material wastage to optimizing energy consumption, ProcessMiner’s solutions are tailored to enhance every aspect of plastics manufacturing.
Sustainable Manufacturing: A Core Objective for the Plastics Industry
In today’s eco-conscious world, sustainability is a crucial objective for any industry, and plastics manufacturing is no exception. By optimizing resource usage and minimizing waste, deep learning and AI technologies are playing a pivotal role in promoting sustainable plastics manufacturing practices. This not only helps in reducing the environmental footprint of plastic production processes but also aligns with the growing consumer demand for eco-friendly products.
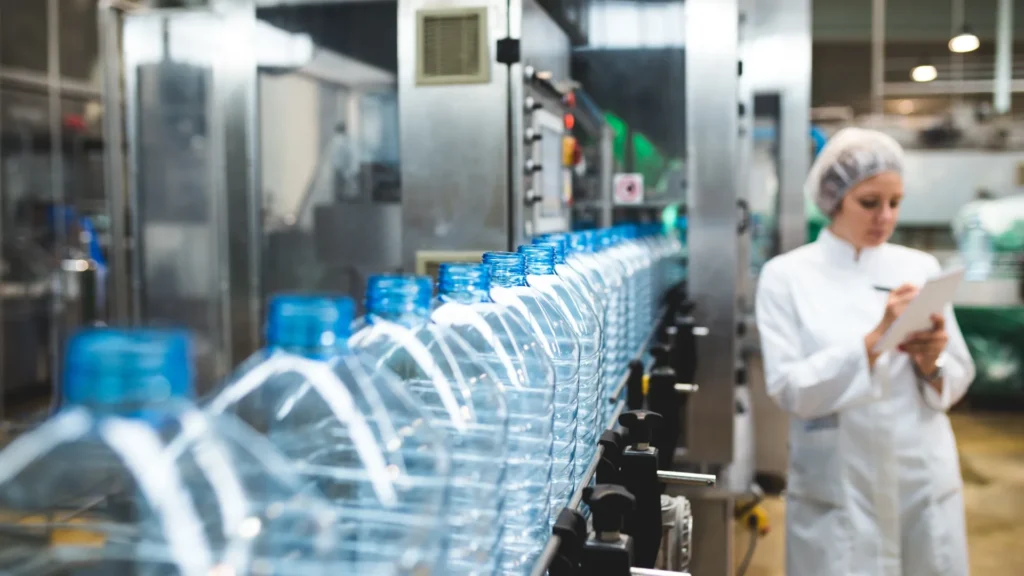
Future Outlook: AI at the Forefront
As we look towards the future, the role of AI and deep learning in plastics manufacturing is poised to grow even more significant. These technologies are expected to become integral components of the manufacturing process, driving innovation, efficiency, and sustainability.
ProcessMiner is committed to being at the forefront of this evolution, continuously enhancing our AI solutions to meet the ever-changing demands of the industry. We invite manufacturers to join us in this journey towards a more efficient, productive, and sustainable future in plastics manufacturing.
Embracing AI for a Sustainable Future in Plastics Manufacturing
The integration of deep learning and AI into plastics manufacturing marks a new era of technological excellence. By embracing these advancements, manufacturers can not only improve their operational efficiency and product quality but also contribute to a more sustainable future. ProcessMiner is proud to be a part of this transformative journey, helping manufacturers harness the power of AI for a brighter, more efficient manufacturing tomorrow.
by Karim Pourak | Aug 31, 2023 | Insights, Wastewater
In the vast tapestry of global challenges, the efficient and sustainable management of water stands out as one of the most pressing. As water scarcity looms and treatment processes grapple with increasing demand and rising costs, the treatment of wastewater becomes a top priority.
No one would argue that wastewater treatment plants are crucial in safeguarding public and environmental health. However, with aging infrastructure and the ever-increasing cost of operations, treatment plants face significant challenges impacting efficiency, effectiveness, sustainability and equipment performance.
In addition to executing regular maintenance, manual sampling and periodic upgrades, developing and executing modernization strategies will be critical in the WWTP’s ability to keep pace with population growth, expanding contamination, wastewater loads and environmental regulations. Advanced technologies, specifically Artificial Intelligence (AI), facilitated by trailblazers like ProcessMiner, are proving to be a game-changer in achieving energy-efficient and sustainable operations, optimizing chemical dosing, and minimizing operational costs.
The Contemporary Challenges of Waste Management in Water Treatment
Traditional Water Treatment Systems:
Historically, wastewater treatment processes are complex and, at times, wasteful. Today, treatment plants typically rely on coagulation, sedimentation, filtration, and disinfection. While these methods are effective, they require significant amounts of chemicals, energy, and other resources.
In addition to typical contaminants, keeping up with the growth of pharmaceuticals, personal care products and other emerging contaminants, which are finding their way into wastewater, poses new challenges. The waste produced in these systems isn’t just about unused water; it’s about the increased use of chemicals, energy, and constant monitoring required to address environmental challenges and potential health risks for consumers.
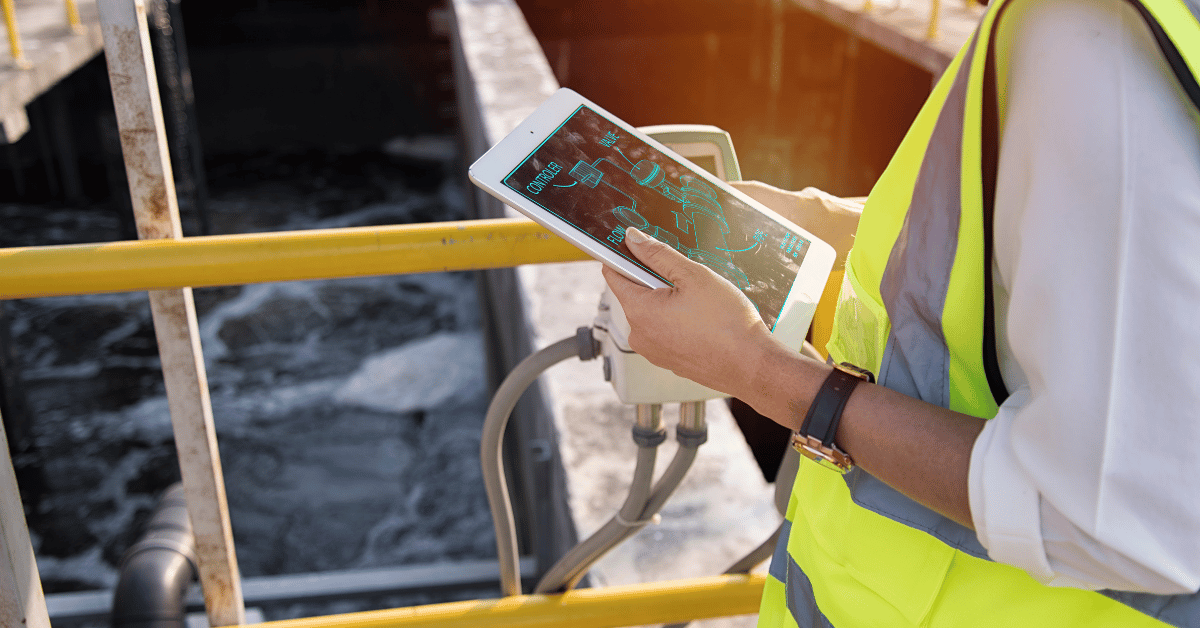
The AI Renaissance: Pioneering Efficient Wastewater Treatment Management
Real-time Optimization with AI:
It will take a combination of technological innovation and infrastructure investment to address these challenges. One emerging technology being applied in wastewater treatment is AI. Integrating automated monitoring systems with the state of AI technology promotes the ability to leverage historical and real-time process data to monitor imbalance events, predict water quality outcomes, and prescribe corrective action to eliminate undesirable outcomes.
In addition, when implemented in a closed-loop architecture, systems can even execute corrective action autonomously without human intervention. Ultimately, reducing the cost of operations (optimizing chemical dosage and energy use) while at the same time improving the consistency of filtrate quality.
Chemical Dosing: Striking the Right Balance with AI
Challenges of Traditional Dosing:
Inconsistent chemical dosing can result in monetary waste and compromise water quality. The right balance is crucial to ensuring effective disinfection and coagulation without overuse.
AI-Driven Precision:
AI, backed by the prowess of Machine Learning techniques, continuously adapts and self-learns from the myriad of historical and in-process data points. This ongoing learning ensures dosing precision while considering factors like water source variability, seasonal changes, and facility-specific requirements.
According to a 2019 study by the Water Research Foundation, facilities that implemented AI-driven solutions reported up to a 20% reduction in operational costs, primarily attributed to optimized chemical dosing and efficient energy consumption.
By integrating AI solutions like those offered by ProcessMiner, facilities can ensure optimal chemical use, high-quality output, and significant cost savings.
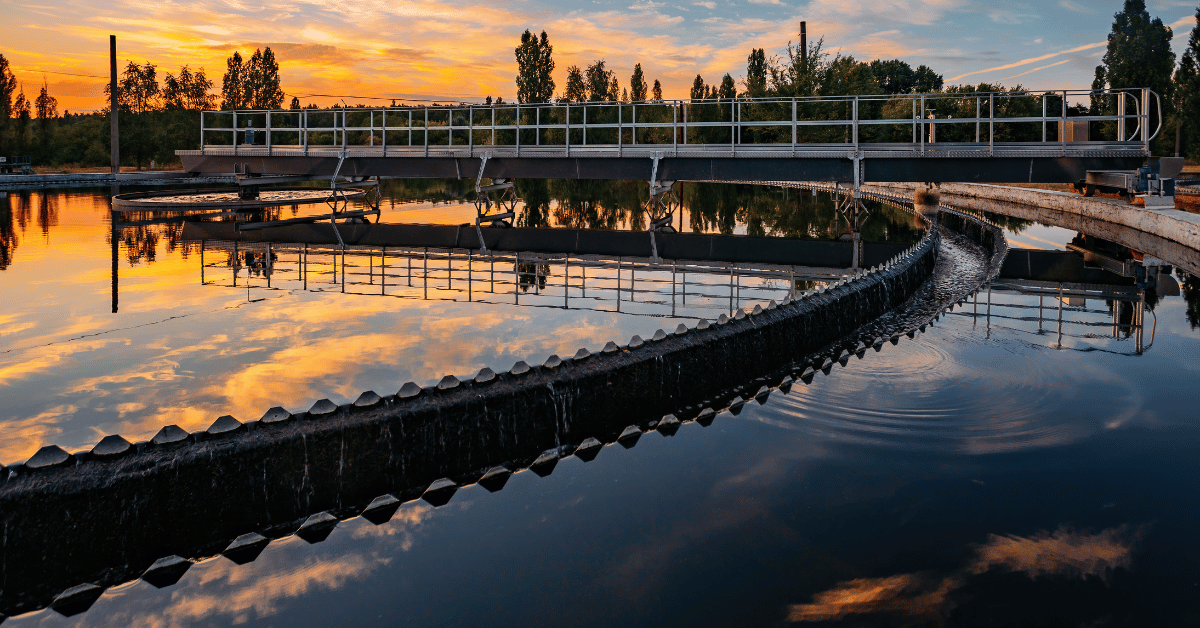
Eco-friendly Wastewater Treatment:
Beyond monetary savings, reducing waste is an environmental imperative. Combining advanced technologies with traditional wastewater management techniques allows treatment facilities to decrease waste production significantly.
A 2020 report by the Environmental Protection Agency (EPA) highlighted that, compared to their non-AI-optimized counterparts over the same period, AI-optimized plants in the U.S. reduced chemical waste disposal into waterways by approximately 15%. This eco-friendly reduction contributes significantly to the preservation of our aquatic ecosystems.
The Future is Clear
The ability to monitor, test, detect and correct imbalance events using only traditional process methods can be inefficient and costly. By addressing the challenges through a combination of technological innovation, infrastructure investment, regulatory compliance, and community engagement, wastewater treatment plants can enhance their efficiency, sustainability, and overall performance. Thus, safeguarding our precious water resources and public health.
If you are ready to elevate your facility’s wastewater treatment process, contact us today. ProcessMiner is ready to partner with you to deliver unparalleled efficiency, waste reduction, and quality assurance. Let’s dive into a more sustainable future together.